Il New York Times ha ufficialmente dato il via libera all’uso di strumenti di intelligenza artificiale per l’editing dei testi, il riassunto delle informazioni, la scrittura di codice e persino la stesura di contenuti. Perché, a quanto pare, i giornalisti umani sono diventati optional. In un’email interna, il quotidiano ha annunciato che il personale editoriale e del prodotto riceverà formazione sull’uso dell’IA, con un nuovo strumento chiamato Echo per riassumere articoli, briefing e altre attività aziendali. Perché leggere tutto un articolo quando puoi avere un riassunto confezionato da un algoritmo?
Autore: Alessandra Innocenti Pagina 4 di 16
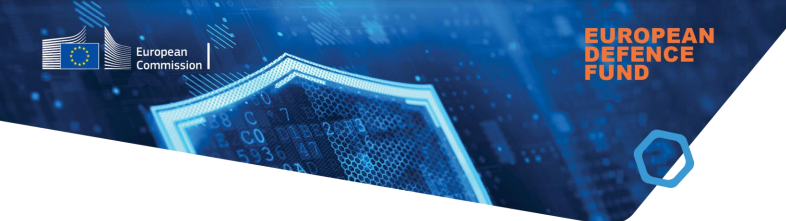
Nel panorama in continua evoluzione della tecnologia e della comunicazione, il progetto AtLaS (Advanced Training for Language and Speech) emerge come un’iniziativa pionieristica volta a trasformare radicalmente il modo in cui interagiamo con i sistemi digitali. Con la partecipazione per l’Italia del professor Roberto Navigli, eminente studioso nel campo dell’elaborazione del linguaggio naturale presso l’Università di Roma “La Sapienza” diag.uniroma1.it, AtLaS si propone di affrontare le sfide più pressanti nel dominio della comunicazione multilingue e dell’intelligence.
Al centro della missione di AtLaS vi è lo sviluppo di sistemi resilienti capaci di gestire il rumore e la complessità derivante dalla molteplicità linguistica. Questo implica l’adozione di tecniche avanzate di addestramento e tecnologie come il denoising, mirate a migliorare la qualità e l’affidabilità delle comunicazioni in ambienti operativi critici. Un aspetto chiave del progetto è la partecipazione all’HLT Challenge, una competizione che stimola l’innovazione nell’ambito delle tecnologie del linguaggio umano, promuovendo soluzioni all’avanguardia per la raccolta e l’analisi delle informazioni.
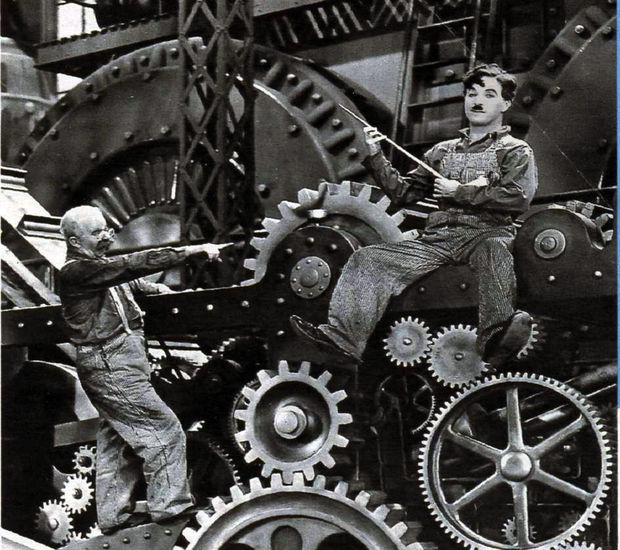
Negli ultimi tempi, l’esperienza di ricerca su Google è stata profondamente trasformata dall’integrazione di AI Overview, una funzionalità che offre una sintesi automatizzata dei risultati. Tuttavia, per molti utenti, questa funzione si è rivelata più un fastidio che un vantaggio, alterando il modo in cui le informazioni vengono presentate e limitando la visibilità dei risultati organici. Fortunatamente, esistono metodi per aggirare questa sovrapposizione di intelligenza artificiale senza dover necessariamente abbandonare Google come motore di ricerca.
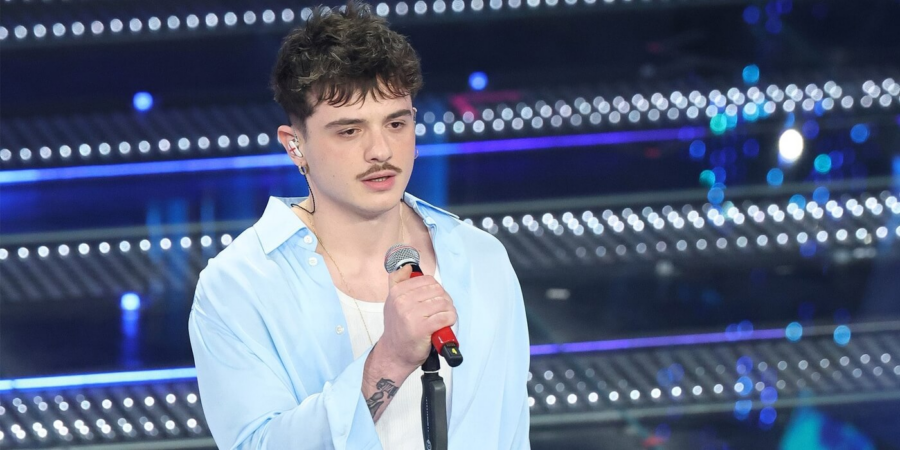
Il 1 Febbraio in pieno Hype Deepseek, la redazione aveva chiesto al nuovo oracolo Cinese, le previsioni per il Festival di Sanremo 2025.
La previsione di Deepseek per Sanremo 2025, basata su artisti già affermati come Achille Lauro, Elodie e Francesco Gabbani, non si è concretizzata a causa di una combinazione di fattori che evidenziano la complessità e l’imprevedibilità del festival. Ecco una sintesi delle ragioni del “fallimento” e del ruolo dei modelli probabilistici.
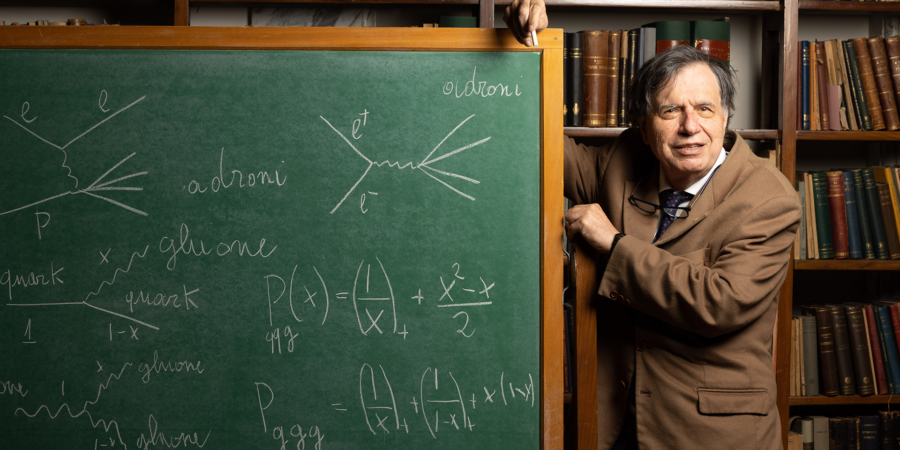

L’Accademia Nazionale dei Lincei e il Gruppo 2003 per la Ricerca Scientifica organizzano un convegno intitolato “Intelligenza Artificiale e Futuro della Ricerca” il 25 febbraio 2025, dalle 10:00 alle 16:30, presso l’Accademia dei Lincei a Roma. L’evento sarà inaugurato dal Premio Nobel Giorgio Parisi e vedrà la partecipazione di esperti come Marco Conti, Niccolò Cesa Bianchi, Giancarlo Fortino, Roberto Navigli e Michele Parrinello. Le discussioni si concentreranno sull’impatto dell’IA in settori come il clima, la biomedicina e l’etica.
Questo convegno rappresenta un’opportunità unica per esplorare come l’IA stia trasformando la ricerca scientifica e affrontando le sfide globali. La presenza di figure di spicco nel campo garantisce approfondimenti di alto livello sulle potenzialità e le implicazioni dell’IA. Per ulteriori dettagli e per partecipare, è consigliabile consultare il sito ufficiale dell’Accademia dei Lincei. Iscriviti qui
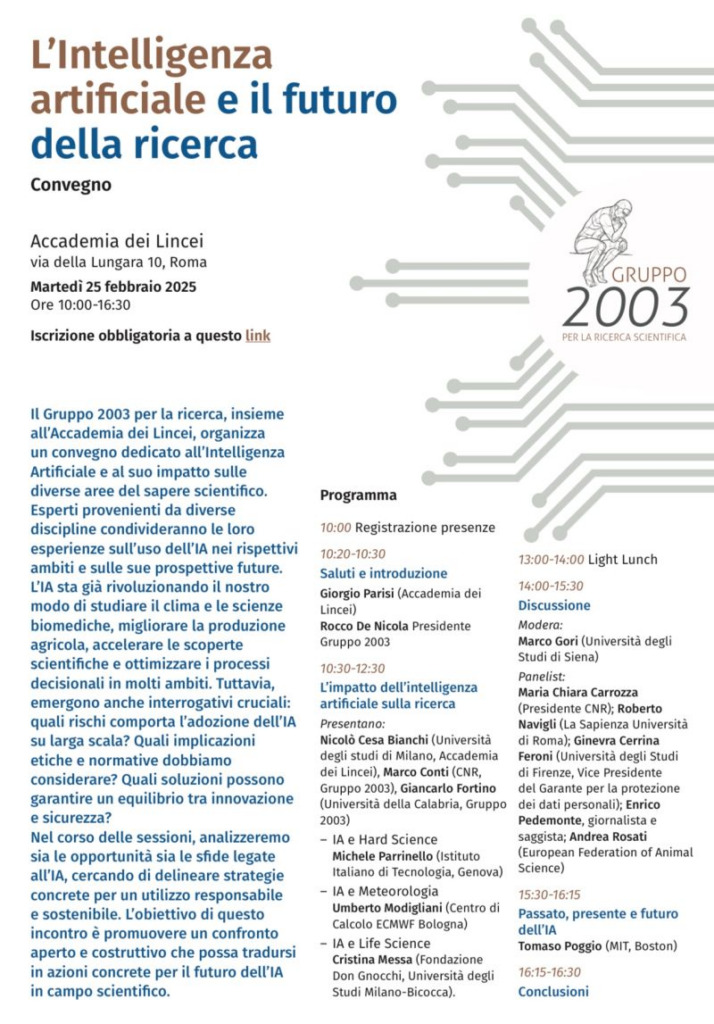
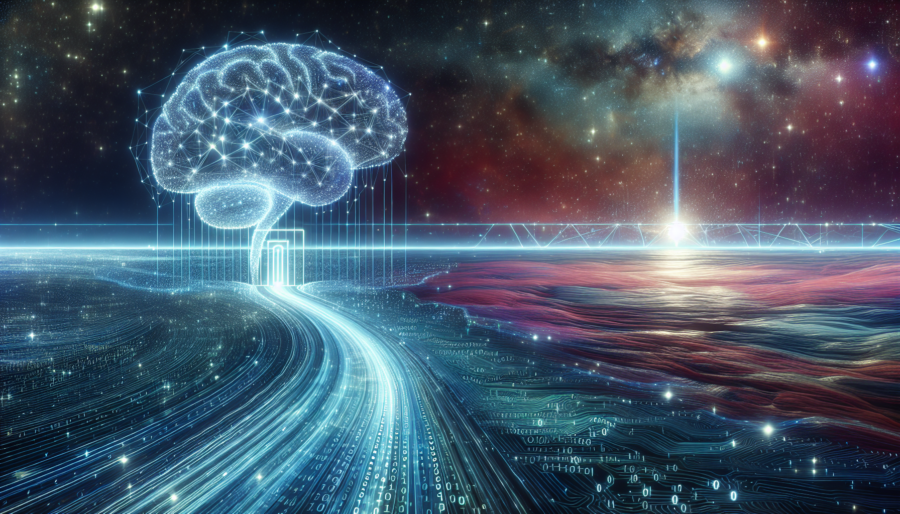
Il concetto di “Private AI” sta guadagnando rapidamente attenzione tra coloro che cercano di bilanciare le potenzialità delle tecnologie di intelligenza artificiale con la necessità di proteggere i propri dati sensibili. Si tratta di un approccio che mira a garantire che l’elaborazione dei dati avvenga senza compromettere la privacy, mantenendo il pieno controllo sui dati stessi. In altre parole, si concentra sull’utilizzo di modelli di AI che operano localmente sui dispositivi dell’utente, piuttosto che fare affidamento su server remoti, come è tipico nei “tradizionali modelli” di intelligenza artificiale basati su cloud.
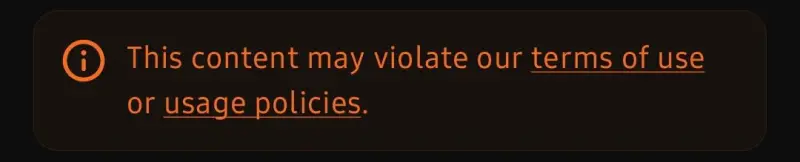
Recentemente, OpenAI ha annunciato la rimozione dei messaggi di avviso su ChatGPT che segnalavano potenziali violazioni dei termini di servizio. Questa decisione rappresenta un cambiamento significativo nella gestione della moderazione dei contenuti sulla piattaforma.
In precedenza, gli utenti di ChatGPT potevano incontrare avvisi che indicavano quando il contenuto generato poteva non essere conforme alle linee guida di OpenAI. Questi avvisi fungevano da promemoria per mantenere le interazioni all’interno dei limiti accettabili e per prevenire l’uso improprio della tecnologia. Tuttavia, tali notifiche sono state oggetto di dibattito, con alcuni utenti che le consideravano intrusive o limitanti per la creatività e la fluidità delle conversazioni.
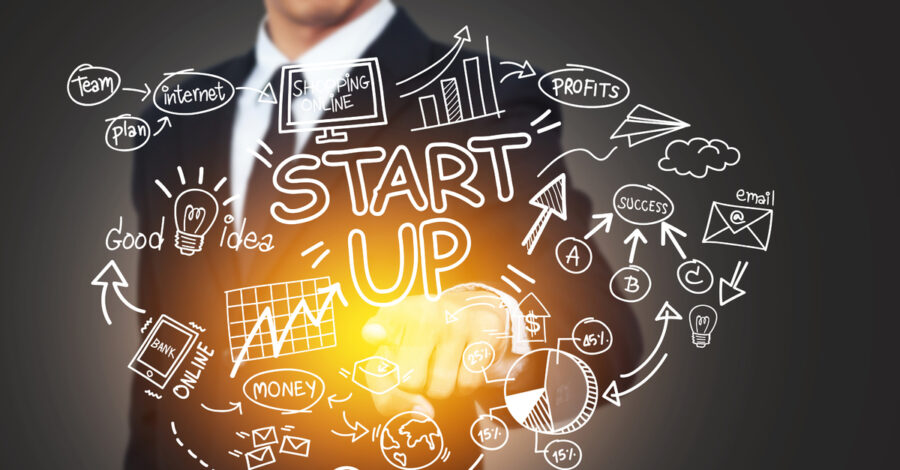
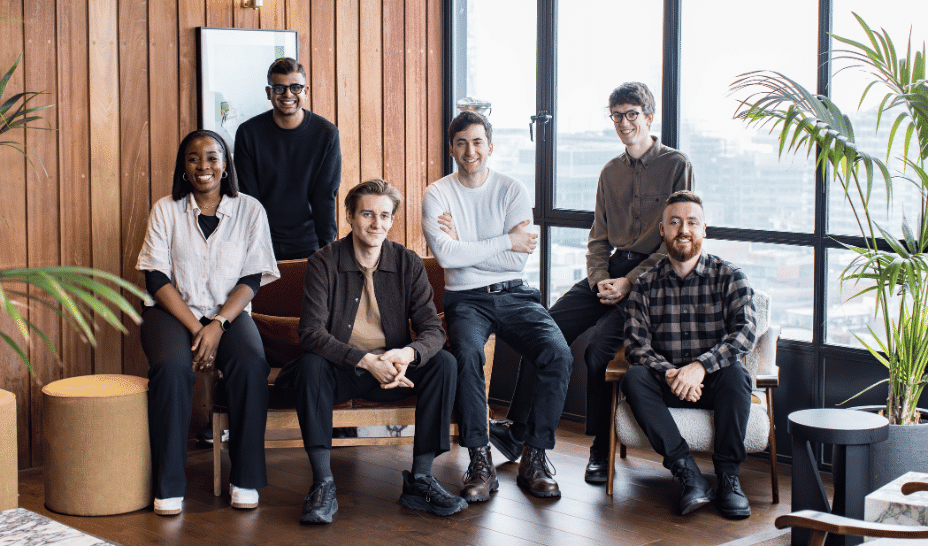
Latent Labs: 50 milioni di dollari per rivoluzionare la progettazione proteica con l’IA
Nel panorama dell’innovazione tecnologica, Latent Labs emerge come una startup all’avanguardia, fondata da Simon Kohl, ex scienziato di DeepMind e co-sviluppatore del programma AlphaFold2. La società ha recentemente raccolto 50 milioni di dollari per sviluppare modelli di intelligenza artificiale dedicati alla progettazione di proteine.
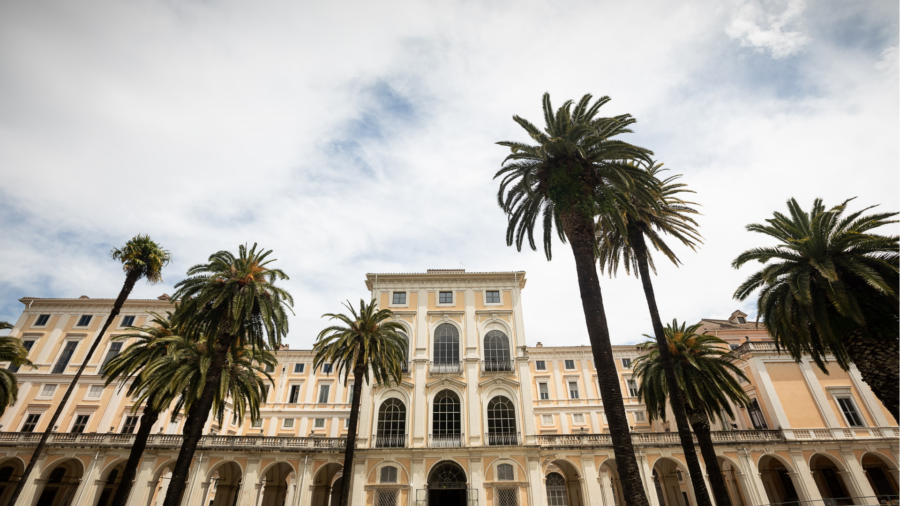

Il 14 febbraio 2025, presso il Palazzo Corsini a Roma, l’Accademia Nazionale dei Lincei ha ospitato una conferenza istituzionale tenuta dal Professor Giorgio Parisi, Premio Nobel per la Fisica nel 2021 e Presidente emerito dell’Accademia. Durante l’evento, Parisi ha affrontato temi cruciali riguardanti l’intelligenza artificiale (IA) e la necessità di un approccio pluralistico per evitare monopoli del sapere.
Parisi ha sottolineato l’importanza di basare l’addestramento dei sistemi di IA su contenuti affidabili e di promuovere la diversità nei modelli di intelligenza artificiale. Secondo il fisico, “l’ideale sarebbe non avere un singolo modello, ma tanti modelli che si confrontano l’uno con l’altro”. Questa pluralità permetterebbe un confronto costruttivo tra diversi sistemi, riducendo il rischio di un monopolio del sapere.
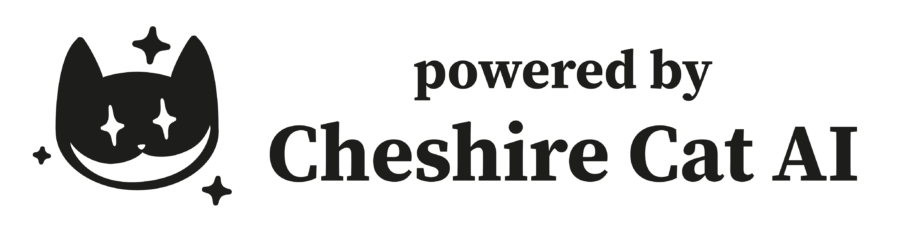
L’intelligenza artificiale sta ridefinendo il nostro modo di interagire con la tecnologia, eppure, nel mare magnum di soluzioni offerte dai colossi tech, un progetto italiano sta facendo parlare di sé per la sua semplicità, flessibilità e potenza. Si chiama Stregatto (Cheshire Cat AI) ed è un framework open source pensato per creare agenti conversazionali e applicazioni di intelligenza artificiale altamente personalizzabili. Ma non è solo un software: è una rivoluzione nel modo di pensare l’IA, nata da una community di sviluppatori e imprenditori italiani.
Anthropic, la startup di intelligenza artificiale fondata dai fratelli italo-americani Dario e Daniela Amodei, sta registrando una crescita impressionante nel settore dell’IA. Secondo un recente rapporto, l’azienda prevede che il proprio fatturato potrebbe raggiungere i 34,5 miliardi di dollari entro il 2027.
Attualmente, Anthropic offre l’accesso ai suoi modelli linguistici avanzati sia attraverso vendite dirette che tramite servizi cloud di terze parti, come Amazon Web Services. Questa strategia ha contribuito significativamente all’espansione dell’azienda nel mercato dell’IA generativa.
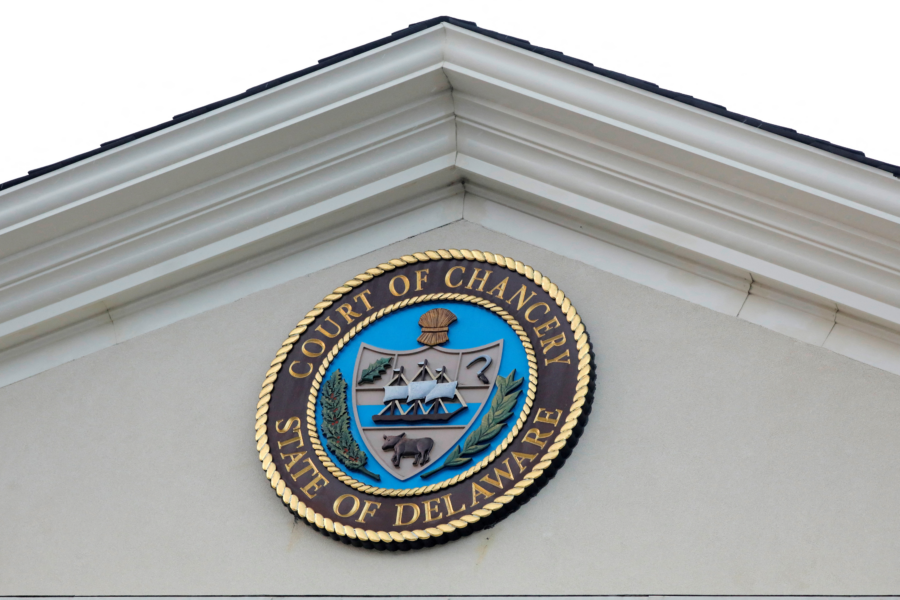
Il 12 febbraio 2025, il giudice distrettuale degli Stati Uniti per il Delaware, Stephanos Bibas, ha emesso una sentenza parziale a favore di Thomson Reuters nella causa per violazione del copyright contro Ross Intelligence, una startup di intelligenza artificiale nel settore legale. Questa decisione rappresenta una pietra miliare nella giurisprudenza relativa all’uso di dati protetti da copyright nell’addestramento di strumenti di intelligenza artificiale.
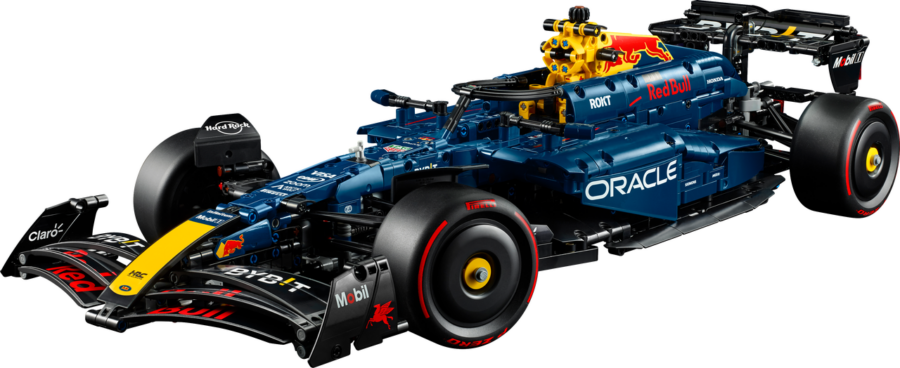
Nel mondo ad alta velocità della Formula 1, dove ogni dettaglio conta e le rivalità sono accese, una nuova battaglia si sta combattendo, ma questa volta fuori dai circuiti. Protagonisti non sono solo i piloti e le scuderie, ma colossi della tecnologia come Oracle e Perplexity. La posta in gioco? La supremazia nel mondo dell’intelligenza artificiale e una partnership da 5 milioni di dollari con uno dei team più vincenti della storia recente: la Red Bull Racing.
Secondo fonti vicine alla vicenda e documenti esaminati da The Verge, Perplexity, startup emergente nel settore delle ricerche AI, era vicinissima a firmare un contratto di sponsorizzazione con Red Bull Racing. Tuttavia, l’accordo è stato bloccato dall’attuale sponsor principale del team, Oracle. La ragione di questo veto risiede in due fattori strategici: Oracle sta cercando di acquisire TikTok, piattaforma su cui anche Perplexity ha messo gli occhi, e sostiene finanziariamente Stargate, un progetto da 500 miliardi di dollari per la costruzione di data center per OpenAI, concorrente diretto di Perplexity.
Dress-1-to-3 è una pipeline innovativa che consente la ricostruzione di abiti 3D separati, fisicamente plausibili e pronti per la simulazione, a partire da una singola immagine catturata in ambienti reali.
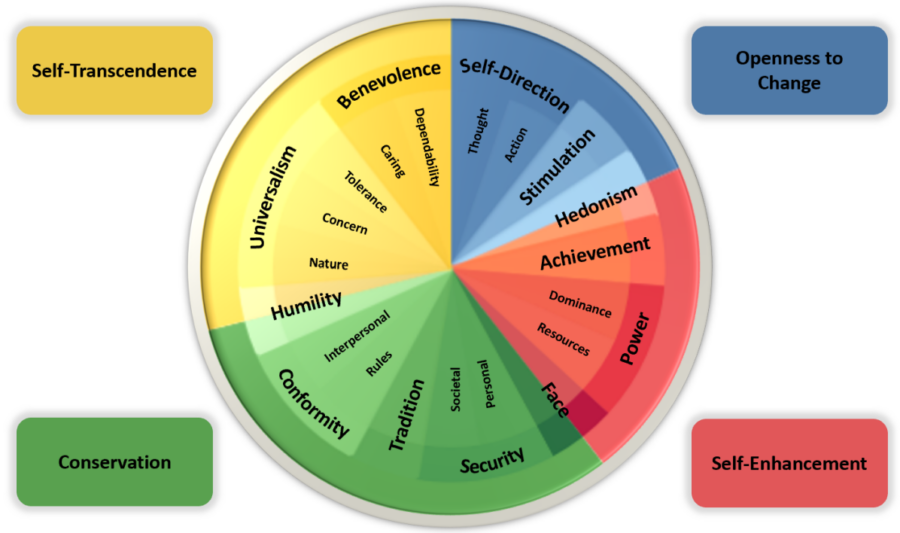
Le AI stanno sviluppando valori propri: una nuova frontiera nell’etica dell’intelligenza artificiale
Un recente studio intitolato “Do LLMs have Consistent Values?” ha evidenziato che i modelli linguistici di grandi dimensioni (LLM) non si limitano a replicare dati, ma mostrano l’emergere di sistemi di valori propri, con “preferenze coerenti” che si rafforzano con l’aumento della scala del modello.
Questo fenomeno implica che LLM statunitensi, come quelli sviluppati da OpenAI, o cinesi, come DeepSeek o Qwen, indipendentemente dalla loro origine o dal fatto che siano open o closed source, tendono a convergere verso gli stessi valori, una convergenza che diventa più pronunciata all’aumentare delle dimensioni del modello.
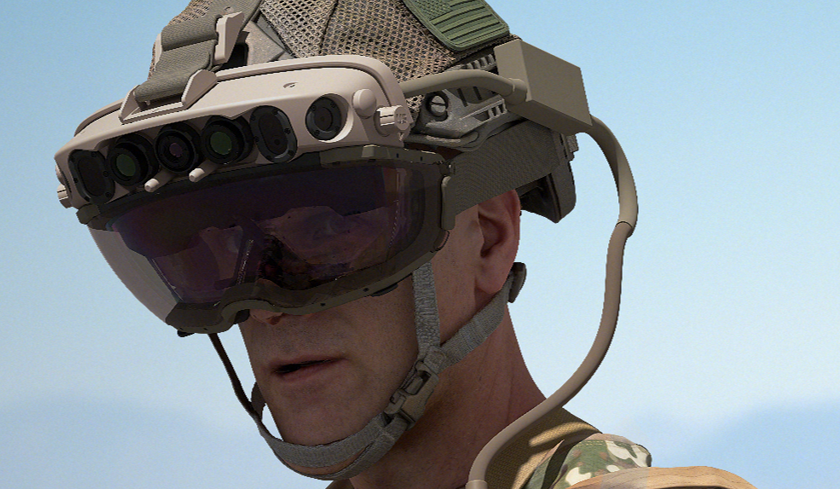
Nel panorama in continua evoluzione della tecnologia militare, Microsoft e Anduril Industries hanno annunciato una partnership strategica per avanzare il programma Integrated Visual Augmentation System (IVAS) dell’esercito degli Stati Uniti. Questa collaborazione mira a integrare la piattaforma software Lattice di Anduril nei visori IVAS, potenziando le capacità dei soldati sul campo di battaglia.
L’IVAS è un sistema progettato per fornire ai soldati una combinazione di realtà aumentata (AR) e realtà virtuale (VR), migliorando la consapevolezza situazionale e supportando il comando di sistemi senza pilota. La piattaforma Lattice di Anduril funge da “sesto senso” digitale, aggregando dati da droni, veicoli e sistemi di difesa per allertare i soldati su minacce imminenti, anche al di fuori del campo visivo. Questa integrazione consente una visione in tempo reale del campo di battaglia, migliorando la sicurezza e l’efficacia operativa.
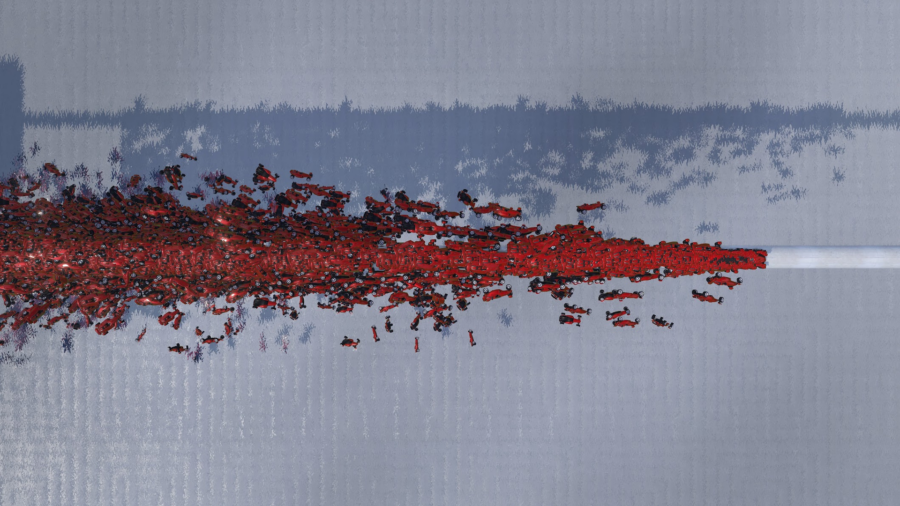
Un nostro lettore di Modena ci ha segnalato YOSH. Ha fatto un lavoro incredibile ci dice Alessandro. Corporation dategli un’occhiata!!
Hi, I’m Yosh and I’m making YouTube videos about a Trackmania AI that I’ve been developing for a few years now. I’ve been playing this game since I was a kid, and I’ve always been curious to know whether it’s possible to determine the optimal way to finish a track using computer science. Since I learned about machine-learning a few years ago, I’ve tried to apply this approach to Trackmania, and have documented my progress in the form of Youtube videos. Between research, programming and editing, these videos take a long time to produce. Any support will help me to spend more time on that in the future 🙂
Nel mondo dei giochi racing, Trackmania è una delle esperienze più affascinanti per gli appassionati di velocità, acrobazie e precisione. La sua natura altamente competitiva e le mappe complesse offrono infinite sfide. Immagina di voler aggiungere un nuovo livello di difficoltà e di innovazione: sviluppare un’Intelligenza Artificiale (AI) per Trackmania, capace di competere o addirittura di superare i giocatori umani. Non solo un’impresa tecnologica, ma anche una possibilità di creare contenuti originali su YouTube, mostrando ogni fase del processo e coinvolgendo una community globale.
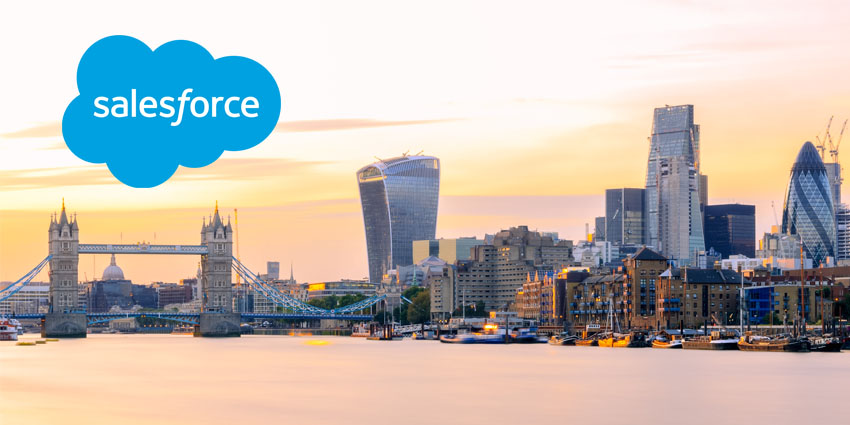
Salesforce, leader globale nel settore del software per la gestione delle relazioni con i clienti (CRM), ha annunciato un investimento strategico di 500 milioni di dollari in Arabia Saudita, focalizzato sullo sviluppo dell’intelligenza artificiale (AI). Questa mossa sottolinea l’impegno dell’azienda nel promuovere l’innovazione tecnologica e nel rafforzare la propria presenza nel Medio Oriente.
Parte integrante di questo investimento è l’introduzione di Hyperforce, l’architettura di piattaforma di Salesforce, nel mercato saudita. Attraverso una partnership strategica con Amazon Web Services, Hyperforce offrirà alle aziende locali una piattaforma scalabile e sicura per gestire le loro operazioni CRM, facilitando l’adozione di soluzioni AI avanzate.
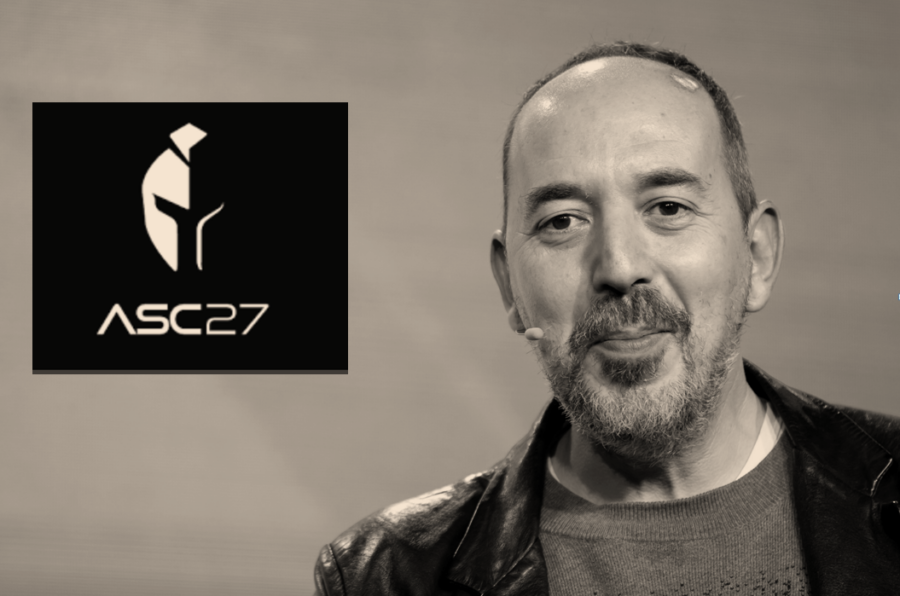
L’Italia entra ufficialmente nella corsa all’intelligenza artificiale avanzata con Vitruvian-1 (V1), un modello sviluppato da ASC27 s.r.l., allenato interamente in Italia e capace di comprendere e rispondere nelle principali lingue europee. Un passo fondamentale per posizionare il nostro Paese e l’Europa tra i protagonisti dello sviluppo tecnologico globale, dimostrando che l’innovazione può nascere anche al di fuori delle grandi multinazionali americane e cinesi.
Vitruvian-1 non è un semplice chatbot, ma un modello AI con capacità di reasoning. A differenza delle tradizionali IA conversazionali, V1 non è progettato per sostenere dialoghi casuali, bensì per risolvere problemi complessi in ambiti come matematica, fisica, chimica e medicina. Può operare sia in modalità CHAT che RAG (Retrieval-Augmented Generation), consentendo agli utenti di sottoporgli documenti e ottenere risposte basate su informazioni specifiche.
Nonostante le dimensioni più contenute rispetto ai colossi da 1500 miliardi di parametri, V1 si distingue per la sua efficienza e la capacità di affrontare problemi con un livello di ragionamento avanzato, arrivando dove modelli più grandi e costosi falliscono. Con i suoi 14 miliardi di parametri, è un compromesso ottimale tra potenza computazionale e sostenibilità economica, rendendolo accessibile per l’utilizzo in ambito industriale ed enterprise.
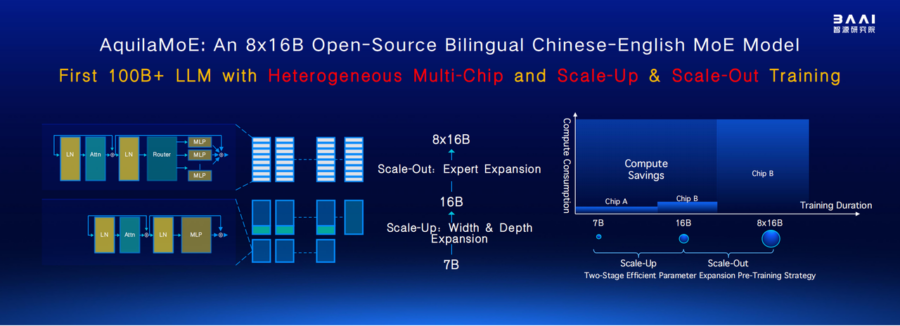
Il Beijing Academy of Artificial Intelligence (BAAI) ha introdotto AquilaMoE, un avanzato modello linguistico basato su una struttura Mixture of Experts (MoE) da 8*16B, progettato per ottenere prestazioni elevate con un’efficienza senza precedenti. Il cuore della sua innovazione è EfficientScale, una metodologia di addestramento a due fasi che massimizza il trasferimento di conoscenza riducendo il fabbisogno di dati e calcolo.
L’approccio Scale-Up permette di inizializzare un modello più grande a partire dai pesi di uno più piccolo, ottimizzando l’uso dei dati e accelerando l’apprendimento. Successivamente, la fase Scale-Out trasforma un modello denso in un modello MoE, migliorando ulteriormente l’efficienza computazionale e la capacità del modello. Queste strategie consentono ad AquilaMoE di superare le limitazioni degli attuali modelli di linguaggio, offrendo prestazioni superiori con minori costi computazionali.
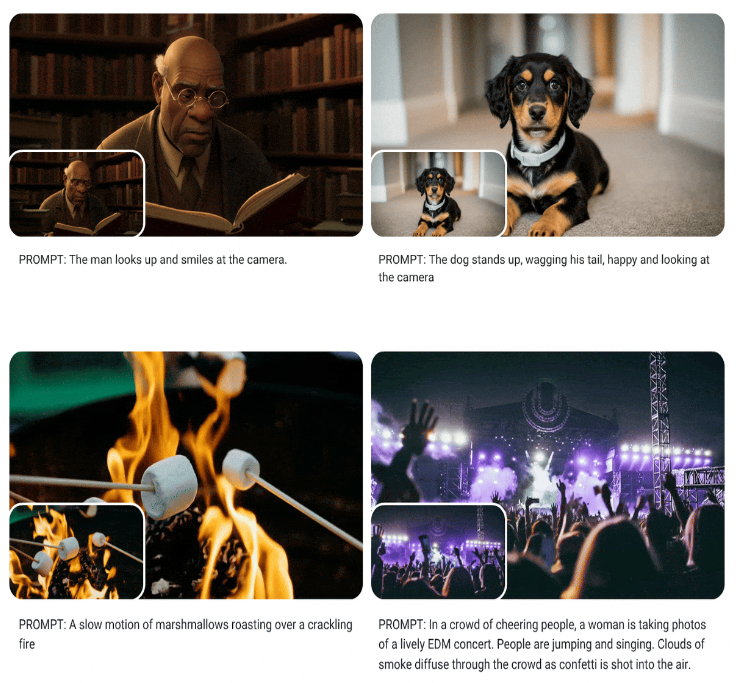
Google ha recentemente introdotto una funzionalità innovativa in Google Workspace che consente agli utenti di generare immagini fotorealistiche di persone utilizzando l’intelligenza artificiale. Questa capacità, inizialmente lanciata in accesso anticipato lo scorso anno, è ora disponibile per tutti gli account Workspace.
La tecnologia alla base di questa funzione è il modello Imagen 3, parte integrante del sistema Gemini di Google. Imagen 3 rappresenta un significativo avanzamento nella generazione di immagini tramite IA, offrendo dettagli eccezionali e una qualità superiore rispetto alle versioni precedenti. Gli utenti possono ora creare immagini fotorealistiche di persone, paesaggi e molto altro, direttamente all’interno dei loro documenti e presentazioni.
Per utilizzare questa funzione in Google Docs, è sufficiente aprire un documento, selezionare il punto in cui si desidera inserire l’immagine e cliccare su “Inserisci” > “Immagine” > “Aiutami a creare un’immagine”. Nel pannello laterale, si può inserire una descrizione testuale dell’immagine desiderata, ad esempio: “Illustrazione gioiosa di una scrivania con un laptop, una tazza di caffè e piante davanti a una finestra con il sole che entra”. Dopo aver fornito la descrizione, l’IA genererà diverse opzioni tra cui scegliere.
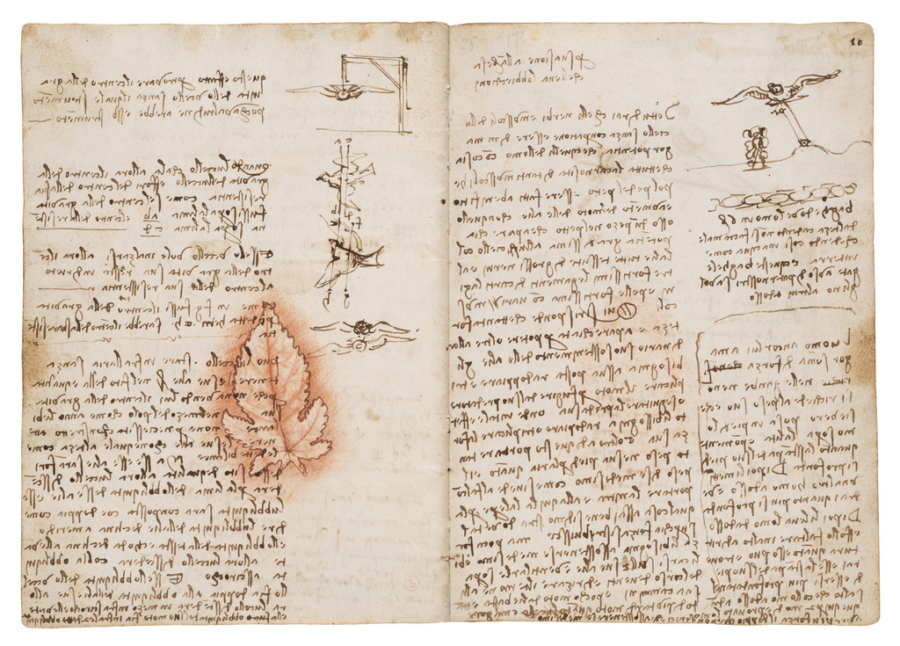
535 anni dopo, il messaggio di Leonardo da Vinci è più vivo che mai: il potere non è nella penna, ma nella mano che la regge. Non nella carta, ma nell’idea che la riempie. E se oggi abbiamo strumenti che sembrano parlare, scrivere, persino pensare, ricordiamoci che essi sono, e sempre saranno, un tributo all’ingegno umano, a quell’eterna fiamma che Leonardo, con la sua carta e la sua penna, contribuì a tenere viva.
Il benchmarking non è solo una questione di numeri, di metriche, di curve che salgono e scendono su grafici colorati. È una lotta contro l’imperfezione, una corsa verso un ideale che forse non esiste, ma che vale la pena inseguire. È il confronto con se stessi, con i limiti del possibile, con la realtà che spesso si rivela più complessa, più ostinata, di quanto si potesse immaginare.
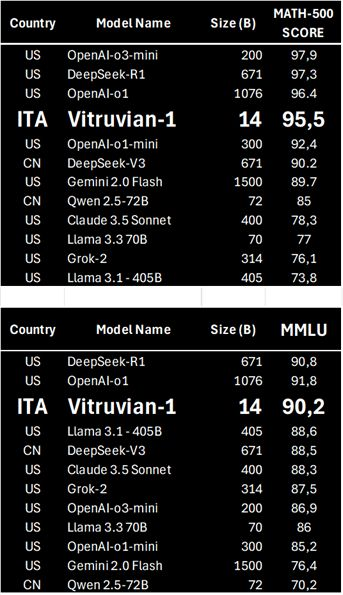
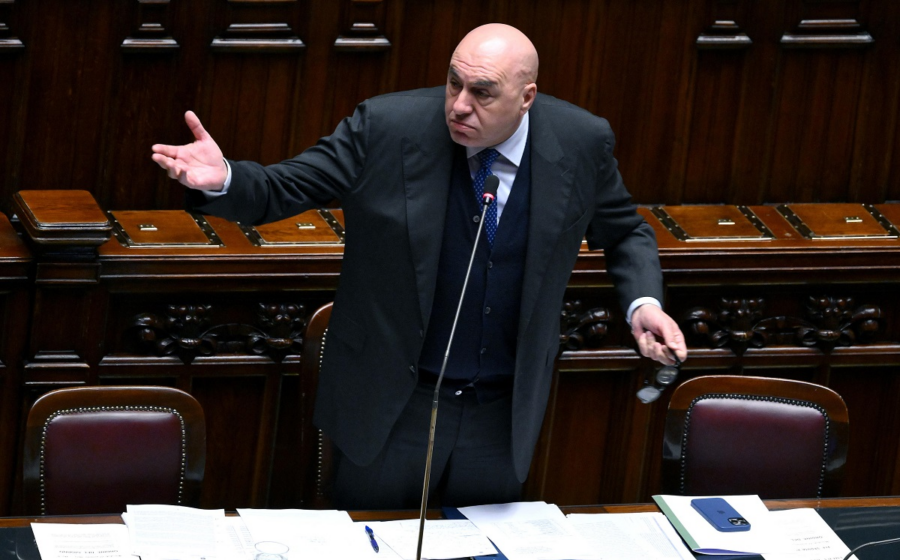
La clonazione vocale basata sull’intelligenza artificiale ha fatto enormi passi avanti negli ultimi anni, permettendo la replica delle voci umane con un realismo sorprendente. Questo progresso, se da un lato ha aperto nuovi orizzonti per l’innovazione tecnologica, dall’altro ha sollevato preoccupazioni riguardo all’uso improprio di queste capacità. Un esempio eclatante di questa problematica è emerso recentemente in Italia, con una truffa ai danni di diversi imprenditori, ingannati dalla voce clonata del ministro della Difesa Guido Crosetto. La truffa ha dimostrato quanto siano facili, per i malintenzionati, sfruttarela manipolazione e ottenere vantaggi illeciti, anche se è stato negato l’uso dell’Ai, vogliamo mettere in guardia i nostri lettori. Non è dato sapere se effettivamente esista il caso in cui è stata usata una voce clonata. Non è certo che fosse la voce di Crosetto; potrebbe essere stata ottenuta tramite un imitatore o una registrazione modificata del ministro, selezionando parole pronunciate in contesti ufficiali o come singola frase.
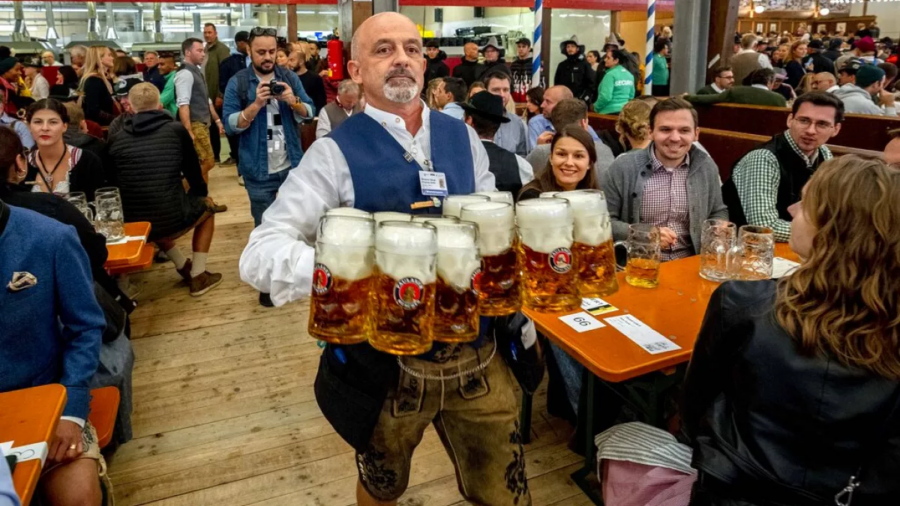
OpenAI continua la sua espansione globale con l’apertura di un nuovo ufficio a Monaco di Baviera, rafforzando la propria presenza in Europa. La scelta della città tedesca non è casuale: Monaco è da tempo considerata la “Silicon Valley della Germania”, un hub di innovazione tecnologica che attrae aziende di primo livello nel settore dell’intelligenza artificiale.
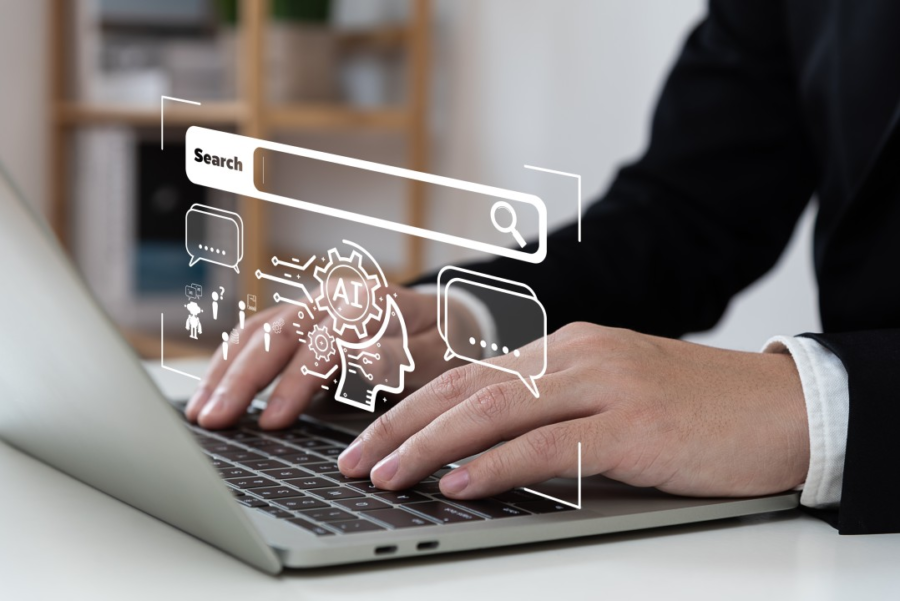
Il crescente utilizzo dei modelli di linguaggio, come GPT-4, in ambiti sempre più complessi come la risoluzione di problemi algoritmici, ha portato alla necessità di un’analisi approfondita delle strategie di prompting per ottimizzare le performance. Un recente studio intitolato “Benchmarking GPT-4 on Algorithmic Problems: A Systematic Evaluation of Prompting Strategies” si concentra su come le diverse tecniche di prompting influenzino l’efficacia di GPT-4 nel risolvere problemi algoritmici. La ricerca ha esplorato vari approcci, evidenziando l’importanza di una progettazione strategica dei prompt per migliorare l’accuratezza e l’efficienza del modello.
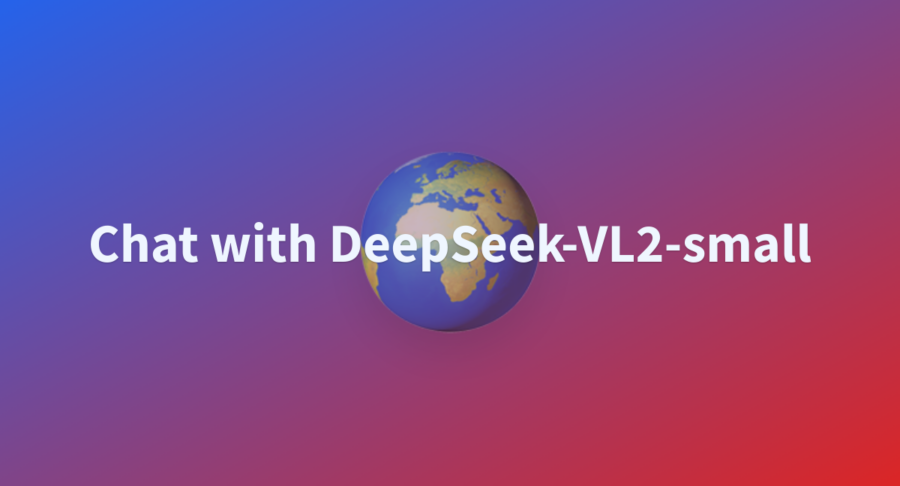
DeepSeek ha recentemente introdotto DeepSeek-VL2-Small, un modello avanzato progettato per l’analisi simultanea di documenti e immagini. Questo modello è ora disponibile per test gratuiti su Hugging Face, offrendo agli sviluppatori l’opportunità di esplorare le sue capacità senza costi iniziali.
DeepSeek-VL2-Small fa parte di una serie di modelli che includono anche DeepSeek-VL2-Tiny e DeepSeek-VL2, con rispettivamente 1,0 miliardi, 2,8 miliardi e 4,5 miliardi di parametri attivati. Questa gamma permette agli sviluppatori di scegliere il modello più adatto alle loro esigenze specifiche, bilanciando complessità e prestazioni.
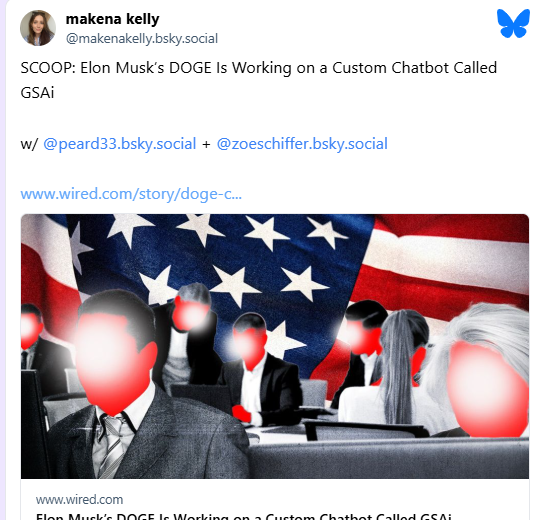
Il settore pubblico si sta sempre più orientando verso l’adozione di soluzioni basate sull’intelligenza artificiale per modernizzare e migliorare l’efficienza nelle operazioni amministrative. Recentemente, un report di Wired ha sollevato l’attenzione su come il DOGE, una figura emergente nel panorama dell’innovazione tecnologica, stia sviluppando un chatbot personalizzato per la General Services Administration (GSA), una delle agenzie più importanti per la gestione dei contratti governativi negli Stati Uniti. Questo chatbot, denominato GSAi, potrebbe rappresentare un salto significativo nel modo in cui vengono analizzati i dati relativi ai contratti e agli appalti pubblici, facilitando una gestione più trasparente ed efficiente.
Secondo le fonti (scoop di Wired), l’obiettivo di DOGE è quello di sfruttare l’intelligenza artificiale per elaborare vasti volumi di dati relativi ai contratti governativi, un’area che tradizionalmente ha comportato un gran numero di complessità burocratiche. Con l’introduzione del GSAi, l’analisi di queste enormi quantità di informazioni potrebbe diventare più rapida e precisa, consentendo di ottenere insight in tempo reale e di ottimizzare le decisioni relative agli appalti e alle procedure di procurement. La visione di DOGE sembra essere quella di trasformare il modo in cui vengono gestiti i dati all’interno della GSA, creando un sistema più agile e dinamico, capace di rispondere alle necessità dei funzionari pubblici e di ottimizzare l’uso delle risorse.
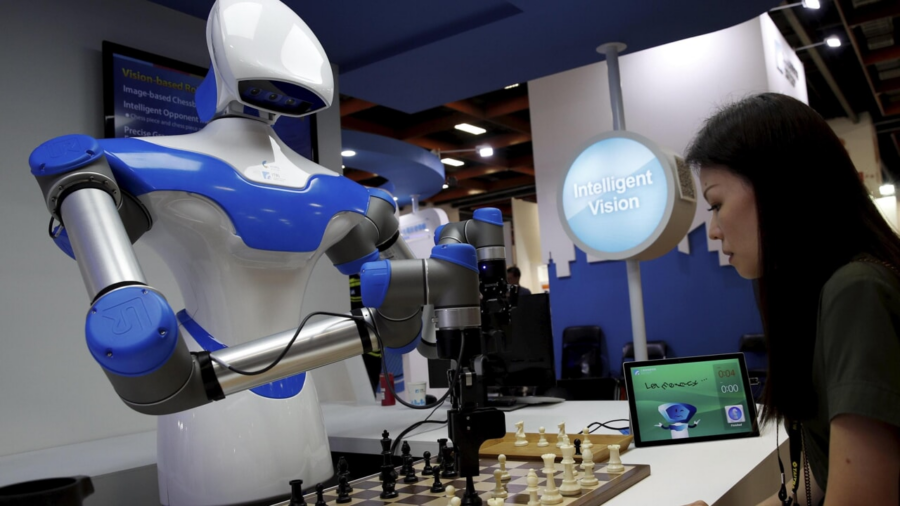
Ecco che Google, dopo aver fatto una timida apparizione nel dicembre scorso con l’accesso a sviluppatori selezionati e tester fidati, ha deciso di aprire il rubinetto: è arrivato Gemini 2.0, la suite di modelli di intelligenza artificiale “più potente” finora creata dal colosso di Mountain View. Ma se vi aspettate una rivoluzione immediata, forse è il caso di rivedere le vostre aspettative.
Gemini 2.0, tanto per iniziare, si presenta con tre varianti. La più robusta, 2.0 Flash, è descritta come “un modello da lavoro, ottimale per compiti di alta frequenza e ad alto volume”, come se le aziende potessero farne un uso intensivo senza battere ciglio. Poi c’è 2.0 Pro Experimental, pensata per gli sviluppatori più affamati di performance nel coding, e infine 2.0 Flash-Lite, la versione economica, ideale per chi vuole spremere ogni centesimo dalla propria carta di credito senza rinunciare alle potenzialità dell’IA. Flash, infatti, costa 10 centesimi ogni milione di “token” per gestire input di testo, immagine e video, mentre la sua versione Lite si ferma a 0,75 centesimi per lo stesso servizio. Non male, no?
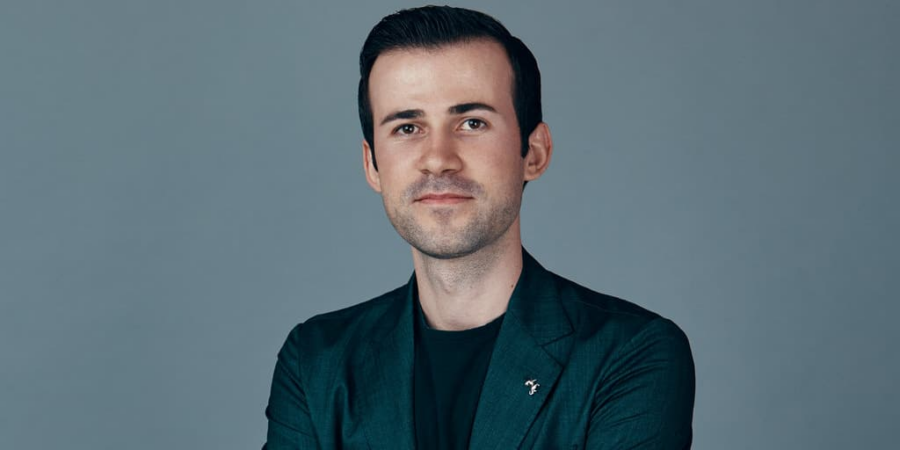
L’Italia si trova di fronte a una delle più grandi opportunità tecnologiche della sua storia. iGenius, una startup italiana fondata da Uljan Sharka, sta costruendo il più potente supercomputer per l’intelligenza artificiale mai realizzato in Europa, grazie a una collaborazione strategica con Nvidia. Un progetto da 1 miliardo di dollari, che potrebbe cambiare per sempre il futuro dell’AI nel nostro Paese e posizionare l’Europa come un player globale in una competizione dominata dagli Stati Uniti e dalla Cina.
Uljan Sharka non è un imprenditore tradizionale. Nato in Albania e trasferitosi in Italia, ha scalato il mondo della tecnologia con un percorso fuori dagli schemi, lavorando come autodidatta fino ad arrivare a San Francisco, il cuore pulsante della Silicon Valley. Lì ha capito una cosa fondamentale: il successo delle aziende tecnologiche è legato alla capacità di pensare in grande e di anticipare il futuro di almeno 20 anni. Con questa visione, ha fondato iGenius nel 2016, partendo da un software di analisi dei dati basato sull’AI, fino ad arrivare a raccogliere oltre 70 milioni di euro e stabilire uffici tra New York e l’Italia.
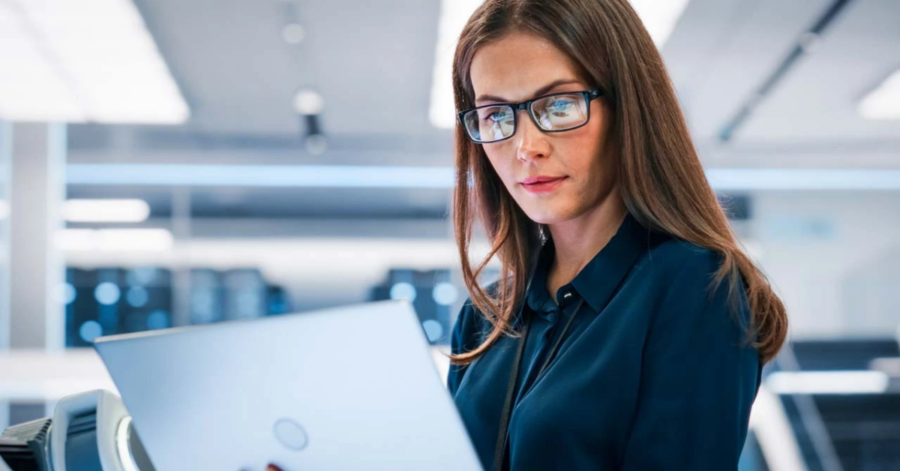
Torna dal vivo l’edizione italiana, moltiplicata in un programma ancora più ricco fatto di tappe di avvicinamento chiamate Stem Days: si parte da Bologna il 21 febbraio con un calendario di appuntamenti per promuovere l’inclusione femminile negli ambiti scientifico-tecnologici.
Dopo il grande successo della prima edizione italiana, accolta entusiasticamente da centinaia di giovani, aziende, istituzioni e i grandi volti del mondo della scienza e della tecnologia, lo STEM Women Congress entra nel suo secondo anno, trasformandosi.
Il format, con un solido heritage internazionale e che ha debuttato per la prima volta lo scorso anno nel nostro Paese, con una trentina di big player, istituzioni e guest star che si sono alternate sul palco, torna, ancora una volta, grazie all’impegno di Orange Media Group, creative content company e di Women at Business, la piattaforma di matching professionale al femminile, costruendo un percorso multiplo su più giornate, chiamate Stem Days, che avrà il suo culmine nel Congresso di Milano il 15 ottobre a Palazzo Castiglioni.
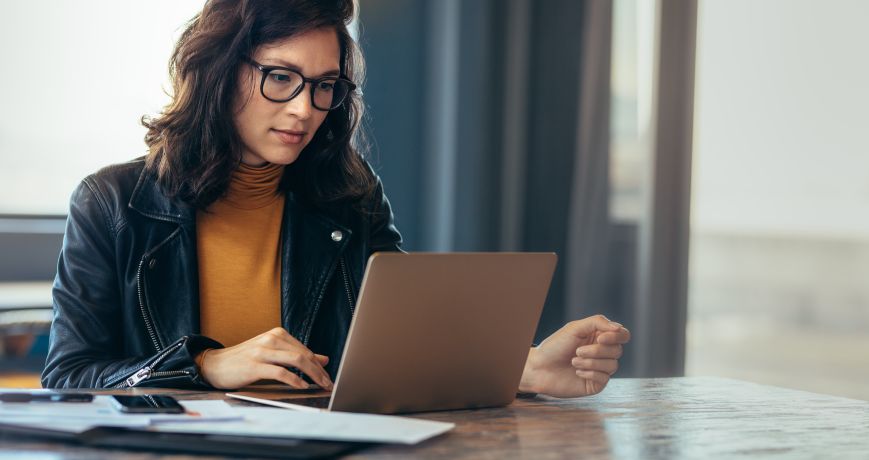
Oracle ha annunciato nuovi Agenti AI basati sui ruoli all’interno di Oracle Fusion Cloud Human Capital Management (HCM) per aiutare i responsabili delle risorse umane e i manager a migliorare la cosiddetta employee experience e ottimizzare la produttività individuale.
Disponibili per i clienti a partire da oggi, i nuovi Agenti AI automatizzano le attività lavorative più noiose in modo da liberare il potenziale delle persone e consentire loro di dedicare il proprio tempo a compiti più significativi.
OpenAI ha annunciato l’introduzione della data residency in Europa per gli utenti Enterprise, Edu e la piattaforma API. Questa novità aiuterà le aziende che operano in Europa a soddisfare i requisiti locali di sovranità dei dati durante l’utilizzo d prodotti OpenAI all’interno delle loro aziende e nello sviluppo di nuove soluzioni basate sull’Intelligenza Artificiale.
Grazie alla data residency, i clienti API potranno scegliere di elaborare i dati in Europa, mentre ai nuovi clienti ChatGPT Enterprise ed Edu verrà data la possibilità di scegliere di conservare i contenuti dei clienti in Europa.
Questo annuncio è il risultato del continuo lavoro per rendere le funzionalità di OpenAI compliant in materia di privacy, sicurezza e conformità dei dati, che oggi supportano centinaia di aziende europee che collaborano con OpenAI.
Google Cambia Rotta sulla Diversità: Stop agli Obiettivi di Assunzione per Gruppi Sottorappresentati
Google ha deciso di interrompere la pratica di fissare obiettivi di assunzione specifici per dipendenti appartenenti a gruppi storicamente sottorappresentati, segnando un cambiamento significativo nella sua strategia di diversità e inclusione. La decisione, comunicata ai dipendenti mercoledì e riportata dal Wall Street Journal, riflette un adattamento delle politiche di Alphabet, la società madre di Google, che ha anche eliminato un passaggio chiave dal suo rapporto annuale. In esso, l’azienda affermava il proprio impegno a rendere la diversità, l’equità e l’inclusione elementi centrali della sua cultura aziendale e a costruire una forza lavoro più rappresentativa degli utenti che serve.
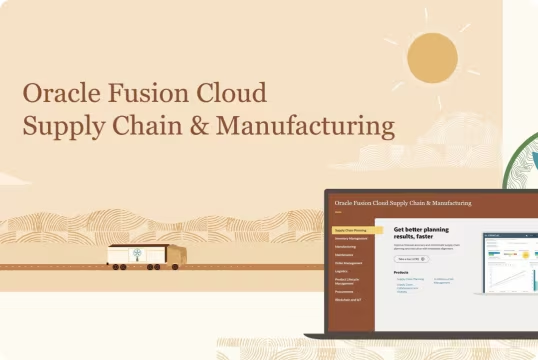
In occasione della tappa di Austin del CloudWorld Tour di Oracle, l’azienda ha presentato due innovazioni chiave che segnano un passo importante nel miglioramento della produttività e dell’efficienza all’interno delle organizzazioni, grazie all’utilizzo avanzato dell’intelligenza artificiale (AI). Queste novità riguardano in particolare la suite Oracle Fusion Cloud Supply Chain & Manufacturing (SCM), destinata a cambiare radicalmente il modo in cui i professionisti della supply chain gestiscono le loro attività quotidiane. L’introduzione di Agenti AI e nuove funzionalità logistiche e di gestione degli ordini promette non solo di semplificare i processi, ma anche di portare un valore significativo in termini di produttività, sostenibilità e customer experience.
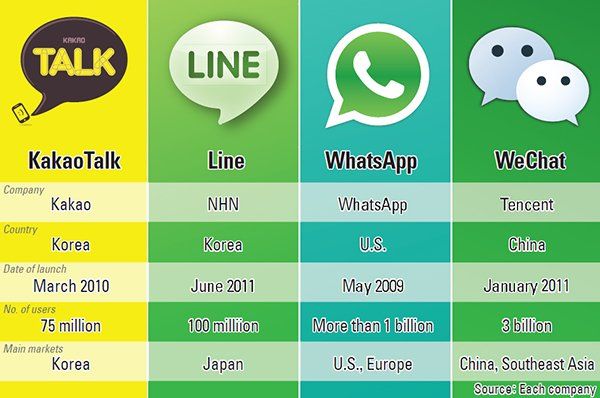
OpenAI ha recentemente annunciato una partnership strategica con Kakao, il principale operatore di app di messaggistica in Corea del Sud, per sviluppare prodotti di intelligenza artificiale (IA) su misura per il mercato coreano. Questa collaborazione mira a integrare la tecnologia avanzata di OpenAI nelle offerte di Kakao, migliorando l’esperienza utente e introducendo strumenti personalizzati basati sull’IA.
Kakao, noto per la sua app di messaggistica KakaoTalk, che detiene una quota di mercato dominante del 97% in Corea del Sud, intende rafforzare le sue capacità nell’IA per competere con rivali locali come Naver. Il CEO di OpenAI, Sam Altman, ha sottolineato l’importanza del mercato coreano per lo sviluppo dell’IA, data la presenza di aziende leader nei settori dei semiconduttori e di internet. Altman ha anche accennato a un potenziale coinvolgimento nel progetto del centro nazionale di calcolo dell’IA della Corea del Sud.
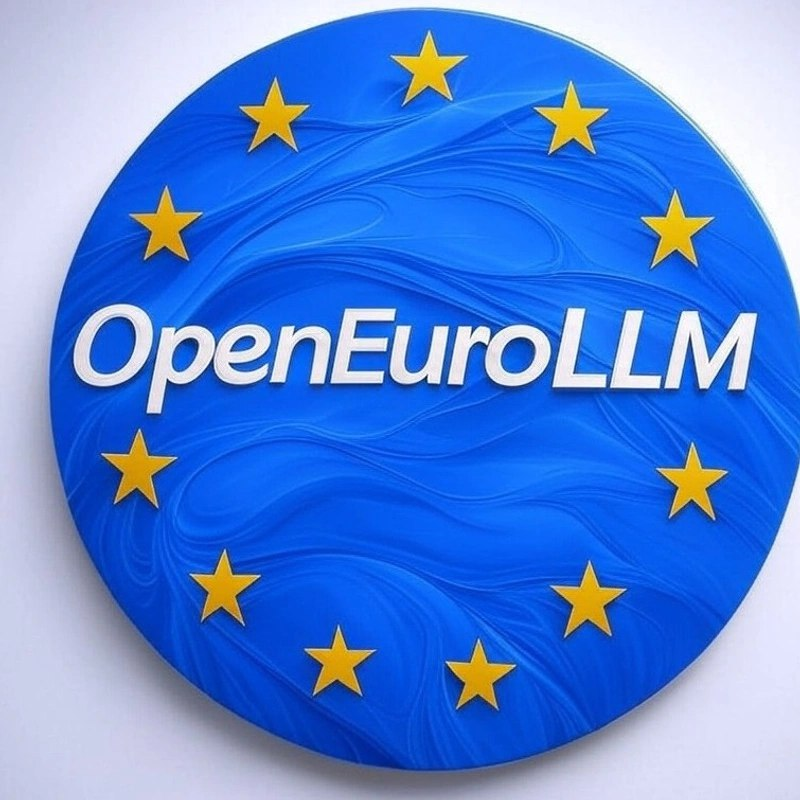
La Commissione ha assegnato oggi il prestigioso marchio della piattaforma per le tecnologie strategiche per l’Europa (STEP) al progetto di intelligenza artificiale (IA) multilingue OpenEuroLLM; si tratta del primo progetto finanziato dal programma Europa digitale a ricevere il marchio STEP.
OpenEuroLLM è un ampio modello linguistico sviluppato dal progetto di ricerca europeo, Open European Large Language Model. È uno sforzo collaborativo per creare un modello linguistico multilingue in grado di comprendere e generare testo in varie lingue europee. Il progetto mira a fornire un modello linguistico più inclusivo e diversificato che possa soddisfare le esigenze degli utenti europei. OpenEuroLLM è progettato per essere un modello altamente scalabile e addestrabile, permettendogli di adattarsi a diversi compiti e applicazioni, come il question-answering, la generazione di testo e la traduzione linguistica.
STEP è un’iniziativa dell’UE volta a rafforzare la competitività industriale europea sostenendo le tecnologie critiche, compresa l’intelligenza artificiale. Il marchio STEP è un marchio di eccellenza dell’UE, che fornisce un accesso semplificato a ulteriori fondi dell’Unione, rafforzando nel contempo il profilo di investimento dei progetti che lo ricevono.
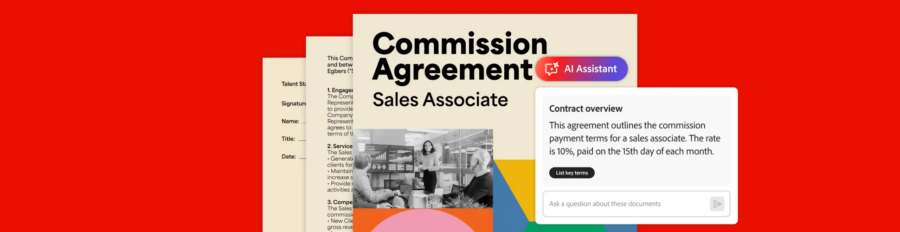
Adobe ha recentemente introdotto nuove funzionalità basate sull’intelligenza artificiale nel suo software Acrobat, per 5$ mese, mirate a semplificare la comprensione dei contratti complessi. Queste innovazioni consentono agli utenti di interagire con i documenti PDF in modo più intuitivo, offrendo la possibilità di porre domande, ottenere risposte immediate e ricevere riassunti dei contenuti. L’AI Assistant di Acrobat è progettato per identificare i contratti all’interno dei documenti e fornire spiegazioni chiare dei termini legali, rendendo più accessibile la comprensione di linguaggi giuridici complessi. Inoltre, è in grado di confrontare fino a dieci versioni di un contratto, evidenziando eventuali discrepanze o modifiche tra le diverse versioni. Queste funzionalità sono attualmente disponibili in inglese su desktop, web e dispositivi mobili, con piani per l’espansione del supporto linguistico in futuro. Per accedere a queste caratteristiche avanzate, gli utenti possono sottoscrivere un abbonamento aggiuntivo al costo di $4,99 al mese, applicabile sia alle versioni gratuite che a quelle a pagamento di Acrobat.
L’integrazione dell’intelligenza artificiale in Acrobat rappresenta un passo significativo verso la trasformazione dell’interazione con i documenti digitali. Secondo Abhigyan Modi, vicepresidente senior di Adobe Document Cloud, questa innovazione cambia radicalmente il modo in cui le informazioni vengono comunicate e consumate, offrendo agli utenti la possibilità di ottenere riassunti, chiarimenti e definizioni in tempo reale. Un motore di attribuzione collega le informazioni alle loro fonti all’interno del documento, garantendo l’accuratezza e prevenendo errori generati dall’IA.
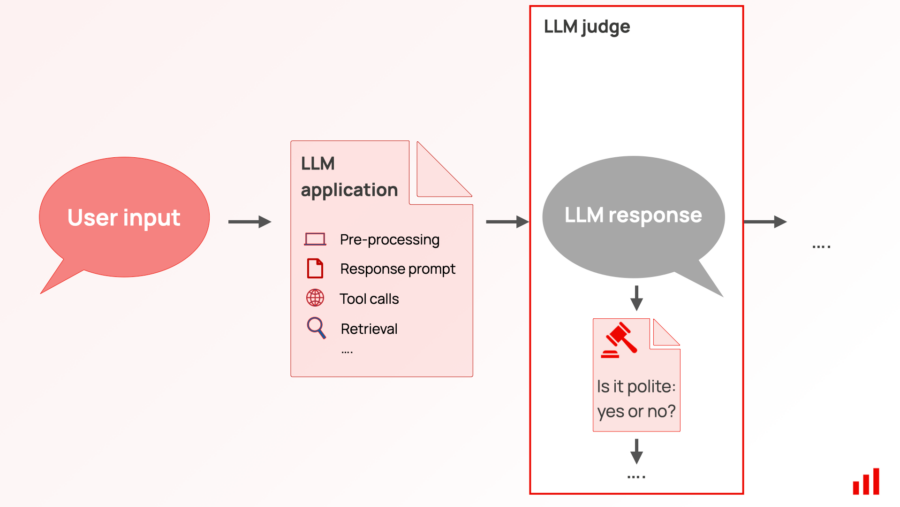
L’avvento dei modelli di intelligenza artificiale basati su Large Language Models (LLM) ha trasformato numerosi settori, dall’elaborazione del linguaggio naturale alla valutazione automatica delle risposte generate dall’IA. Tuttavia, un problema fondamentale è la difficoltà di strutturare il ragionamento in modo che il processo di valutazione sia coerente, trasparente e interpretabile. Il paper Learning to Plan & Reason for Evaluation with Thinking-LLM-as-a-Judge affronta proprio questa sfida, introducendo EvalPlanner, un algoritmo che separa la fase di pianificazione della valutazione da quella dell’esecuzione, ottimizzando così il processo di giudizio dell’LLM.
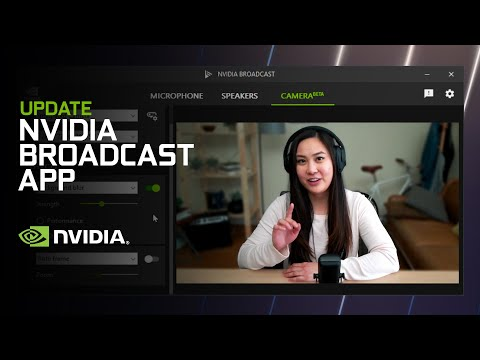
Nvidia continua a spingere i limiti dell’intelligenza artificiale, questa volta migliorando l’esperienza video con il suo software gratuito Nvidia Broadcast. L’ultimo aggiornamento introduce nuove funzionalità AI che promettono di elevare la qualità delle trasmissioni video e audio per streamer, creatori di contenuti e professionisti del lavoro remoto.
Le nuove feature integrate sono progettate per affrontare alcune delle problematiche più comuni delle webcam e dei microfoni standard. “Studio Voice” utilizza l’intelligenza artificiale per migliorare la qualità dell’audio, filtrando i rumori indesiderati e ottimizzando la voce dell’utente. “Eye Contact” è una funzione particolarmente interessante che regola automaticamente lo sguardo, facendo apparire l’utente come se stesse sempre guardando direttamente la fotocamera, anche quando non lo è. Questa tecnologia è particolarmente utile per chi registra video o partecipa a riunioni online, eliminando la distrazione di uno sguardo vagante.